The healthcare industry produces an astonishing amount of data every day approximately 137 terabytes! From medical devices and imaging systems to genomic research and EHRs, this data holds a lot of value that the healthcare industry can rely on. For instance, imaging data analysis can enhance diagnostics, with AI analyzing X-rays and MRIs to detect conditions early.
Genomic data can help identify genetic risks and tailor treatments. Even structured EHRs and unstructured doctor notes feed predictive models, improving care decisions and streamlining patient pathways. By compiling these datasets, healthcare institutions get access to unexplored insights that can boost patient outcomes, cut costs, and improve healthcare delivery efficiency. Let’s explore more in this area and see how big data is implemented in different areas within healthcare.
Sources of Big Data in Healthcare
Big data comes from various sources, each contributing uniquely toward healthcare intelligence and improved patient outcomes. These data streams include:
- EHRs: Detailed patient information, including demographics, treatment histories, and lab results.
- Medical Imaging: Data from X-rays, MRIs, and CT scans contribute massive and high-resolution datasets.
- Wearable Devices and IoT Sensors: Data from smartwatches, fitness trackers, and IoT-enabled devices.
- Genomic Data: Data from genomic sequencing practices.
- Clinical Trials: Data from clinical trials, including patient outcomes, medicine efficacy, and side effects.
Comprehensive healthcare datasets can also be sourced from open-source databases such as Observational Health Data Sciences and Informatics, the CDC, WHO, and data.gov.
However, accessing raw data is just the first step. Professional data extraction service providers can help extract and compile healthcare data from these sources. They can also help process, clean, organize, and standardize this data, ensuring it is analysis-ready and compatible with healthcare analytics platforms.
How Big Data is Transforming Healthcare?
Here are the most impactful big data implementation areas in the healthcare industry.
1. Clinical Decision Support Systems (CDSS):
CDSS uses healthcare data analytics to examine a patient’s data against millions of similar cases instead of solely relying on standard treatment guidelines. As a result, they require volumes of historical data from diverse sources like patient histories, imaging results, and lab reports. This availability of big data ensures these systems provide personalized, evidence-backed recommendations for diagnoses and treatments.
2. Healthcare Claim Denial Management:
According to a report published by the American Medical Association (AMA), healthcare claim denial rates reached an all-time high of 11% in 2022 (considering the 1700 hospitals that were evaluated). For patients, denied claims often mean delayed or unaffordable care, and for healthcare providers, they can result in lost revenue and administrative burdens.
However, with a big data analytics solution for healthcare, you can process thousands of claims and identify the root cause. For instance, the reason for a claim’s denial could be incomplete patient demographics, a policy/coverage mismatch, filing errors, or duplicate claims. This data equips you to manage claim denials more proactively, enabling you to flag data discrepancies on time.
3. Virtual Healthcare Assistants
Heard of Babylon Healthcare, the well-known digital-first virtual healthcare assistant? Although no longer operational, it set a precedent for using AI-powered analytics to deliver health assessments and telemedicine services. Similar virtual healthcare platforms can harness big data, such as patient histories, prescription patterns, and clinical outcomes, to generate valuable insights and provide highly personalized care.
Chatbot VAs such as UCHealth’s Virtual Assistant “Livi” can also monitor users’ vitals, such as glucose patterns, heart rate, etc., through IoT devices. Based on this information, they can alert, provide reminders, and even predict insulin requirements (for those with diabetes).
4. Robotic Surgical Assistance
Robotic surgery systems rely on big data to enhance precision and improve surgical outcomes. These systems can plan and execute procedures with accuracy by integrating patient-specific data such as imaging scans, previous surgical results, and anatomical details. Using this data, they can also anticipate potential challenges during operations where actual anatomy cannot be viewed through scans and surgeons have to go in without much information at hand.
5. Healthcare Business Intelligence (BI)
Datasets containing information on patient demand, seasonal variations, and patient flow trends can be used to gain healthcare BI insights. For instance, big data analytics on seasonal variations, such as flu outbreaks, enable hospitals to forecast spikes in inpatient admissions. This allows them to schedule additional staff or allocate resources such as ICU beds and equipment well in advance. Similarly, analyzing patient flow data across departments helps identify bottlenecks, such as delays in emergency room transfers or discharge processes.
6. Remote Patient Monitoring (RMP)
Remote patient monitoring systems rely on big data from wearables, IoT devices, and EHRs and provide continuous patient health insights. For instance, a wearable device monitoring a cardiac patient can transmit heart rate and rhythm data to a cloud-based solution for medical data management. If irregularities are detected, the system immediately notifies the caretaker, allowing for timely intervention. This has positioned RPM as a highly sought-after service, with more than 70.6 million patients using RPM systems in the US alone.
7. Medicine Repurposing
Repurposing is particularly helpful when traditional medicine development faces challenges like high costs, lengthy timelines, and limited patient populations for trials. That’s where big data analysis on existing medicines, new trials, chemical properties, and patient outcomes can help you identify new therapeutic uses for existing medications. This approach also shortens the time required for development as Phase I safety trials are often bypassed since the medicine’s safety profile is already established.
8. Predicting Patient Readmissions
According to a systemic review conducted by the NIH, the average readmission cost within a 30-day time frame is around USD 16,037.08. Predicting patient readmissions can be greatly optimized using big data analytics to analyze discharge summaries, demographic data, and treatment histories. ML models can analyze these datasets to identify high-risk patients, allowing you to implement preventive measures such as follow-up appointments or home-based care.
9. Epidemiological Surveillance and Pandemic Response
Epidemiological surveillance benefits immensely from big data. By integrating data from EHRs, social media, and environmental sensors, epidemiological surveillance enables real-time tracking of disease outbreaks. This information helps health officials identify hotspots, predict the spread of diseases, and plan interventions. The CDC’s spontaneous outbreak surveillance is a classic example. It has a dedicated Office of Public Health Data, Surveillance, and Technology (OPHDST) that provides easy access to disease-specific surveillance data.
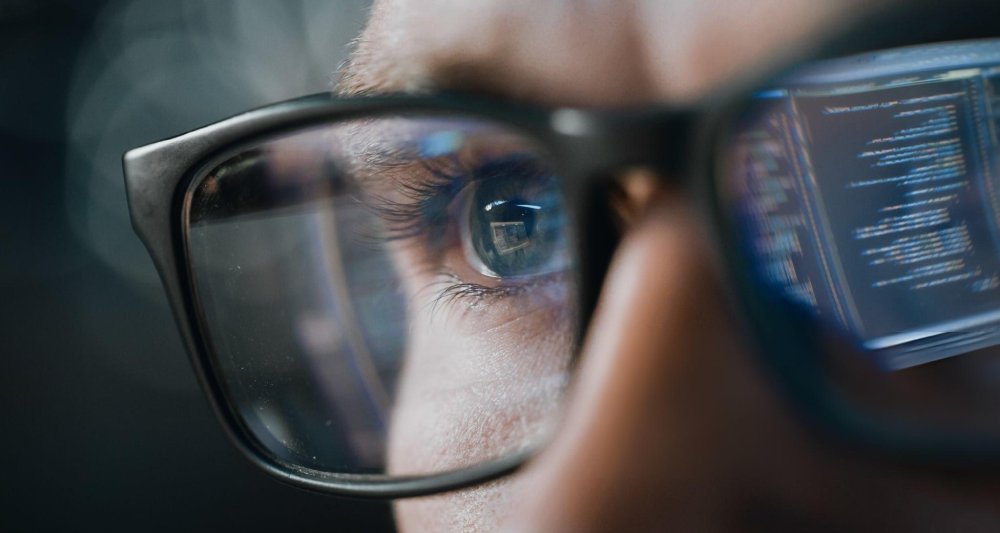
Making Big Data Work: Driving Technologies and Systems
Big data alone cannot improve medical research and overall healthcare outcomes. It must be used cohesively with the right technologies. Here are some of the enablers of big data:
1. AI and ML in Healthcare
AI and ML are central to big data analytics. With these technologies, you can:
Analyze imaging data, such as X-rays and MRIs, necessary to detect early signs of diseases like cancer or neurological disorders.
Process historical EHR data to predict outcomes such as patient readmissions, enabling preventive care.
Convert unstructured data, like physician notes, into structured formats for analysis.
2. Cloud Computing
This technology provides the scalable infrastructure needed to store, process, and share vast healthcare datasets securely. Additionally, cloud-based solutions for medical data management allow you to process data from multiple sources, such as wearable devices, imaging systems, etc., in real time. Another significant benefit is the guaranteed and prompt data backups and quick recovery in case of system failures, which is critical for maintaining continuity in patient care.
3. IoT and Wearable Technologies
In combination with IoT, wearable technologies ensure a consistent flow of real-time patient data, such as heart rate, glucose levels, or blood pressure. When integrated with big data systems, they facilitate:
- Remote patient monitoring
- Population-level insights after analysis
- Early detection of potential health issues
- Better medication adherence
- Behavioral health tracking
- Data Integration and Analytics Platforms
These platforms are designed to unify, process, and analyze diverse datasets from multiple sources while addressing the complexity of fragmented healthcare data structured (e.g., EHRs, lab results) and unstructured (e.g., doctor notes, imaging data). This approach allows you to benefit from higher interoperability in healthcare systems.
While the technologies mentioned above form the backbone of big data analytics, effective implementation depends on coordinated efforts across all stakeholders hospitals, researchers, policymakers, technology providers, and even patients.
Ensuring Collaboration for Effective Big Data Implementation
Here is how each stakeholder can contribute to successfully implementing big data in healthcare.
- Healthcare Institutions must ensure proper data augmentation, invest in scalable infrastructure, and prioritize compliance with data privacy regulations.
- Medical Researchers and Technologists are crucial in building tools that process, analyze, and derive actionable insights from complex datasets.
- Policymakers and Regulators create frameworks to maintain ethical data usage and security while encouraging innovation in big data technologies.
- Patients can contribute by consenting to data sharing, using wearable devices, and embracing technology-driven healthcare solutions.
End Note
Big data sure has the potential to redefine medical research, patient care, and healthcare delivery. However, realizing this potential requires more than just access to healthcare data it demands a proper infrastructure and the integration of a suite of technologies such as AI, ML, cloud, and frameworks like IoT, etc.
Successfully implementing big data in healthcare also requires collaboration among stakeholders hospitals, researchers, policymakers, and technology providers ensuring that data is used ethically and efficiently. With the right systems, strategies, and safeguards, big data can drive innovations that improve patient outcomes, reduce costs, and pave the way for a healthier future.
Author Bio :
Nathan Smith is a Technical Writer at TechnoScore with extensive knowledge of software documentation, API guides, and user experience. Mastering HTML, CSS, JavaScript, and tools like JIRA and Confluence, Nathan’s expertise covers Application Development, Software Engineering, AI/ML, QA Testing, Cloud Management, DevOps, and Staff Augmentation (Hire mobile app developers, hire web developers, and hire full stack developers, etc.). Outside of work, he enjoys traveling and food blogging.